Home | Idris I. Haidar
My wife and I spent most of our honeymoon visiting several shopping malls near our first residency. We lived in a gated community, but it is rather isolated and the surroundings looked like a sub urban area. The “nearest” favorite shopping mall even takes 30 minutes drive with a common traffic. Worst traffic can take one hour drive. The same applies for visiting the nearest decent hospital, market, train station, and other mandatory public facilities.
It’s a cool place to live for introverts though. Sadly, I’m not an introvert. I still like to visit public places and meet people.
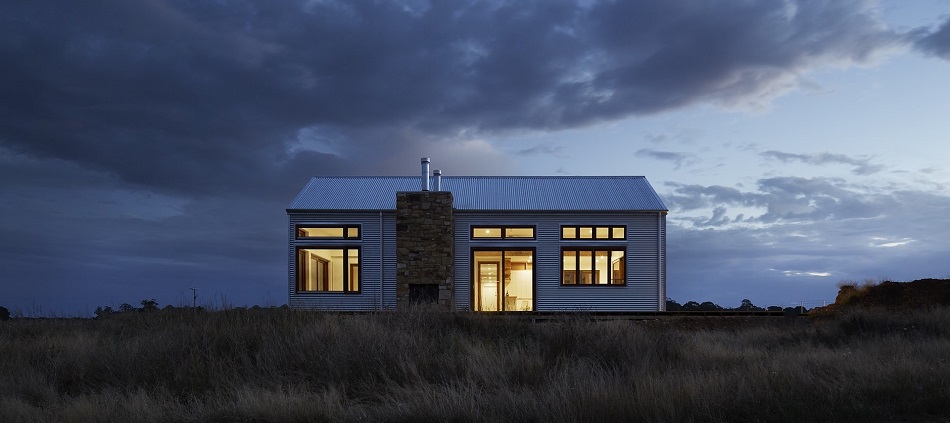
Source: LeeSecurity.com
We are running a campaign to offer a certain product to a customer. An offer obviously may not be accepted at the first trial, so each customer can be offered the same product several times up to, say, six times, or until he/she accepted the offer.
From the marketer’s perspective, if they send an offer to a customer and then the customer rejects it, it can be counted as a “failure”. So the marketer can “fail” multiple times before their offer is accepted by a customer, which will be a “success” for them.
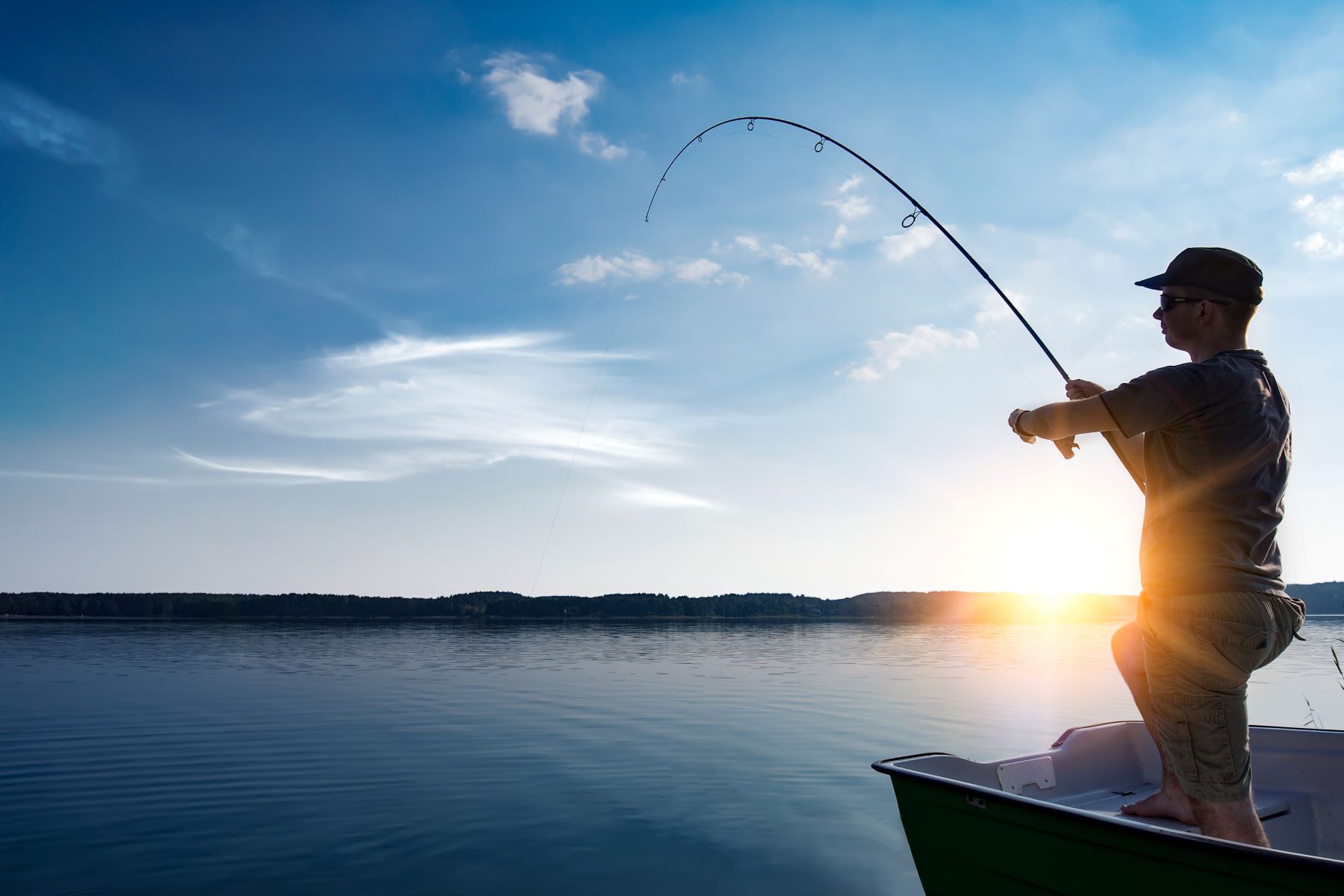
Source: freshwatervacationrentals.com
Repeat customers can be considered as the key players of a settled and profitable business. Repeat customers help us to get more purchases without needing to acquire new ones. Repeat customers are easier to gain a trust from. Repeat customers even help us to acquire more first time buyers.
Acquiring new customers might cost higher than making the existing ones to purchase more. Even so, the latter seems to be more difficult. Business actors need to formulate when is the right time to appeal an existing customer to make his/her next purchase.
Given a customer has made a purchase once, for example, how long one should wait for him/her to make the second one. By estimating when the second purchase will be made, it might be easier to measure how challenging it is to expect more purchase from someone.
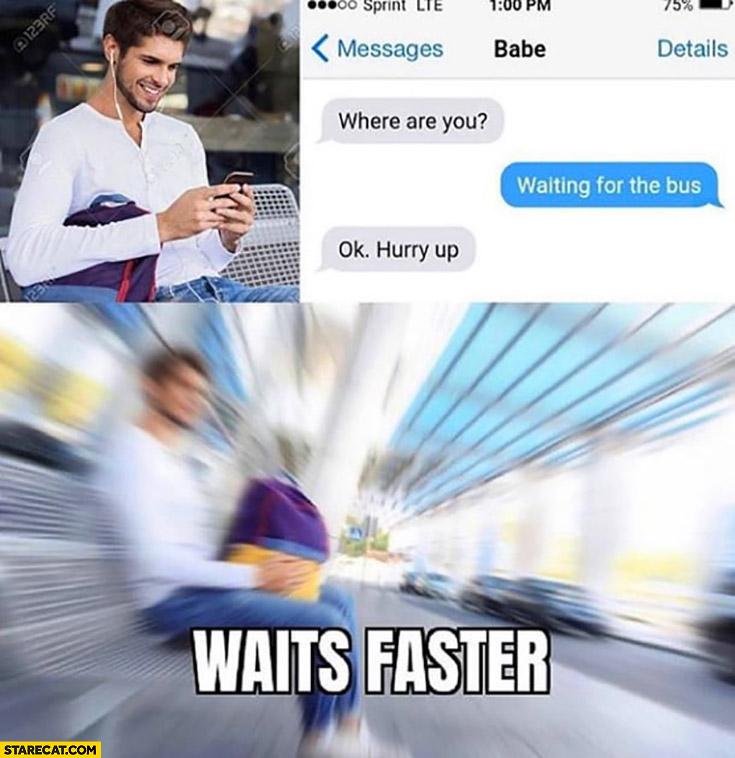
Source: starecat.com
Let’s simulate this question with a Survival Analysis.
There are several purposes of applying dimensionality reduction into a dataset. We often times need to communicate our high-dimensional dataset into a simpler visualization, either in 2D or 3D. Sometimes our model training seems very slow because of the number of features or the size of the data. Sometimes we just need to drop some unnecessary details and noise from our data.

Source: Wiktionary
If you’re someone who have been taking benefits from dimensionality reduction, you might need to revisit the implementations you’ve done for a while. In many cases, this technique can be very advantageous, but in some others, it turns out that there would some pitfalls in applying PCA for certain problems.
Let’s talk about Principal Component Analysis (PCA) as a matter of problem framing. Suppose that you’d like to reduce our dataset dimensionality in order to reduce the training duration of your linear regression model. You’re planning to do so because previously your model got relatively unacceptable training duration. The model seemed also to only work well in the training set.
While implementing PCA, you suddenly recall about how reducing features from \(n\) to \(k\) features could simplify the model and help avoiding overfitting. You also wonder how PCA could help you find the so-called “most important features” to predict your label. You then proceed your PCA implementation and finally obtain the principal components.
In retail and e-commerce industries, customers can share their experiences after making purchases in a certain platform. Their reviews usually can also be seen by other customers. Customers might not decide whether to buy a product before seeing other customers’ review first. Thus, review can be considered as one way for customers to express their view as a platform’s user to the other users.
A review does not only describe the product, the service, and the transaction experience, but also the behaviour of the customer him/herself. Getting know about customers would be a good step to know their painpoints and whether those affect their behaviour.
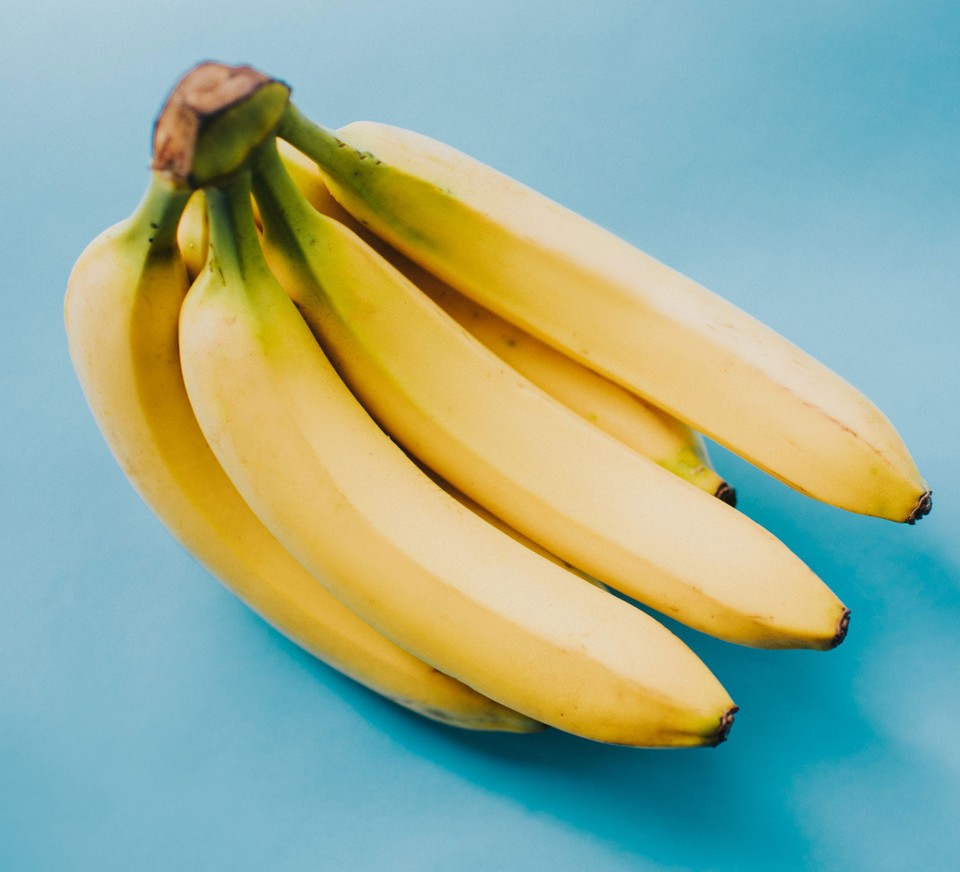
Source: bbcgoodfood.com
Consider the most prominent aspect of a review: rating (e.g. rating that describes how satisfied you are in a 1-to-5 star range). In terms of writing reviews, there are some behaviours that can be empirically expected from customers. If a seller offers a certainly bad product or service then someone buys his product and gives bad review, the upcoming reviews tend to be similar.
Let’s see another case. If a buyer gets a bad experience purchasing from a certain seller, either it is about the product or the service, will the upcoming reviews from the same buyer but for other different sellers be similar? Suppose that someone has given 1-star rating for a purchase. What is the probability that he gives another 1 star for his next purchase? Or what is the probability of him giving another star?
How does a previously given review affect another upcoming review by the same person?
I write tiny stuffs about data science, analytics, and ML/AI. Sometimes I also write about tech career in general and also fatherhood, maybe.
I hope you find some of my writings interesting.